No. 1 (2017)
DOI https://doi.org/10.18799/24056529/2017/1/110
Modelling and control of crystallization process
Batch crystallizers are predominantly used in chemical industries like pharmaceuticals, food industries and specialty chemicals. The nonlinear
nature of the batch process leads to difficulties when the objective is to obtain a uniform Crystal Size Distribution (CSD). In this study, a linear
PI controller is designed using classical controller tuning methods for controlling the crystallizer outlet temperature by manipulating the inlet
jacket temperature; however, the response is not satisfactory. A simple PID controller cannot guarantee a satisfactory response that is why an
optimal controller is designed to keep the concentration and temperature in a range that suits our needs. Any typical process operation has
constraints on states, inputs and outputs. So, a nonlinear process needs to be operated satisfying the constraints. Hence, a nonlinear controller like
Generic Model Controller (GMC) which is similar in structure to the PI controller is implemented. It minimizes the derivative of the squared error,
thus improving the output response of the process. Minimization of crystal size variation is considered as an objective function in this study. Model
predictive control is also designed that uses advanced optimization algorithm to minimize the error while linearizing the process. Constraints are
fed into the MPC toolbox in MATLAB and Prediction, Control horizons and Performance weights are tuned using Sridhar and Cooper Method.
Performances of all the three controllers (PID, GMC and MPC) are compared and it is found that MPC is the most superior one in terms of settling
time and percentage overshoot.
Keywords:
Batch crystallization, Crystal size distribution, Crystal size variation, Optimal control, Constraints, Model predictive control
Downloads
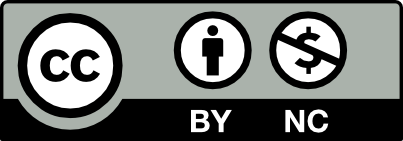