No. 5 (2016)
DOI https://doi.org/10.18799/24056529/2016/5/83
Modeling the adsorption of benzeneacetic acid on CaO2 nanoparticles using artificial neural network
The present work reported a method for removal of benzeneacetic acid from water solution using CaO2 nanoparticle as adsorbent and modeling
the adsorption process using artificial neural network (ANN). CaO2 nanoparticles were synthesized by a chemical precipitation technique. The
characterization and confirmation of nanoparticles have been done by using different techniques such as X-ray powder diffraction (XRD), high
resolution field emission scanning electron microscope (HR-FESEM),transmittance electron microscopy (TEM) and high-resolution TEM
(HRTEM) analysis. ANN model was developed by using elite-ANN software. The network was trained using experimental data at optimum
temperature and time with different CaO2 nanoparticle dosage (0.002–0.05 g) and initial benzeneacetic acid concentration (0.03–0.099 mol/L).
Root mean square error (RMS) of 3.432, average percentage error (APE) of 5.813 and coefficient of determination (R2) of 0.989 were found for
prediction and modeling of benzeneacetic acid removal. The trained artificial neural network is employed to predict the output of the given set of
input parameters. The single-stage batch adsorber design of the adsorption of benzeneacetic acid onto CaO2 nanoparticles has been studied with
well fitted Langmuir isotherm equation which is homogeneous and has monolayer sorption capacity.
Keywords:
Feed forward neural network, Single-stage batch, Benzeneacetic acid, CaO2 nanoparticles, Adsorption
Downloads
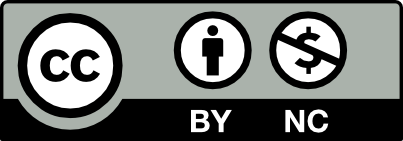